Machine learning is a branch of artificial intelligence (AI) that uses algorithms and statistical models to enable systems to automatically improve their performance on a specific task over time without being explicitly programmed. It involves using large datasets and sophisticated algorithms to learn from patterns, relationships, and insights in data.
Marketing refers to creating, communicating, delivering, and exchanging value offerings for customers, clients, partners, and society. It involves understanding customer needs and preferences, developing products and services to meet them, and promoting them through various channels to attract and retain customers.
Marketing increasingly uses machine learning to enhance customer experience, drive engagement, and improve business outcomes. By leveraging large volumes of customer data, marketers can develop more accurate and personalized marketing strategies catering to each customer’s needs and preferences. This results in more effective targeting, better customer segmentation, and improved decision-making. Additionally, machine learning can help businesses predict customer behaviour, detect fraud, and identify patterns that human analysts may miss. As such, machine learning is becoming an integral part of modern marketing, and its use is expected to grow significantly in the coming years.
Applications of Machine Learning in Marketing
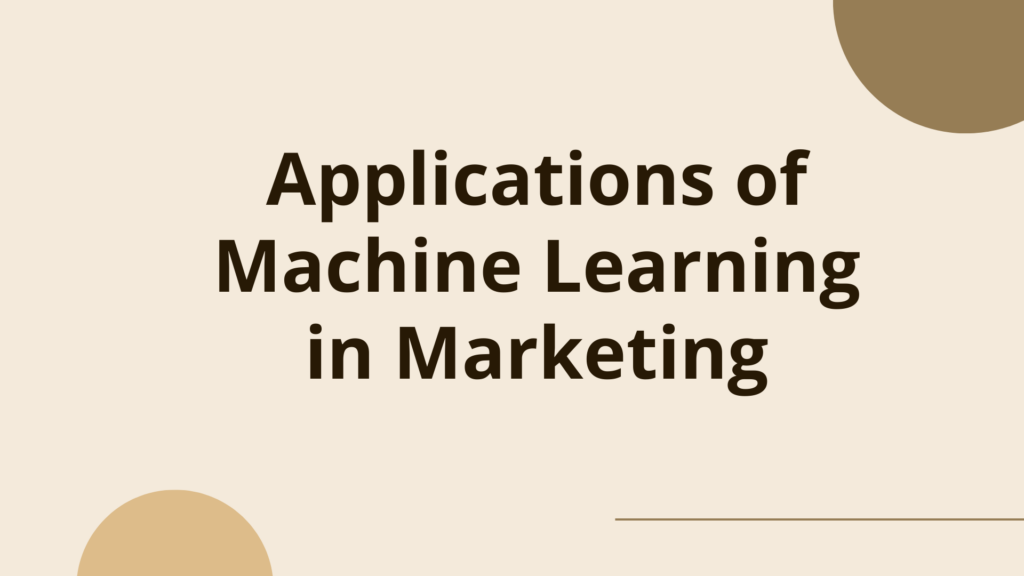
Personalized Marketing:
Personalized marketing involves tailoring marketing messages and offerings to individual customers’ unique needs and preferences. Machine learning algorithms can analyze customer data to identify patterns and behaviours, which can be used to personalize messaging and product recommendations. This approach can result in higher engagement and conversion rates and increased customer satisfaction.
Customer Segmentation:
Customer segmentation involves dividing customers into groups based on shared characteristics or behaviours. Machine learning algorithms can analyze customer data to identify patterns and group customers into segments based on demographics, buying behaviour, and preferences. This approach can enable businesses to target specific segments with more relevant marketing messages and offers, leading to increased customer loyalty and retention.
Predictive Analytics:
Predictive analytics involves using historical data to make predictions about future outcomes. Machine learning algorithms can analyze past customer behaviour to predict future behaviour, such as the likelihood of making a purchase or churn. This approach can help businesses to anticipate customer needs and take proactive steps to retain customers and drive revenue growth.
Churn Analysis:
Churn analysis involves identifying customers at risk of leaving and taking steps to retain them. Machine learning algorithms can analyze customer data to identify churn-related factors, such as low engagement or dissatisfaction with a product or service. This approach can enable businesses to proactively retain at-risk customers by offering personalized promotions or improving product features.
Fraud Detection:
Fraud detection involves identifying and preventing fraudulent activity. Machine learning algorithms can analyze large volumes of data to detect patterns and anomalies indicating fraudulent activity, such as unusual account activity or suspicious transactions. This approach can help businesses to prevent financial losses and protect customer data.
Recommendation Engines:
Recommendation engines involve using customer data to provide personalized product or content recommendations. Machine learning algorithms can analyze customer behaviour to identify products or content that may be interesting and provide personalized recommendations based on that data. This approach can help businesses to increase customer engagement and drive revenue growth.
Sentiment Analysis:
Sentiment analysis involves analyzing customer feedback to understand their attitudes and emotions towards a product, service, or brand. Machine learning algorithms can analyze customer feedback, such as social media posts or customer reviews, to identify sentiment patterns and areas where improvements can be made. This approach can help businesses to improve customer satisfaction and brand reputation.
Benefits of Machine Learning in Marketing
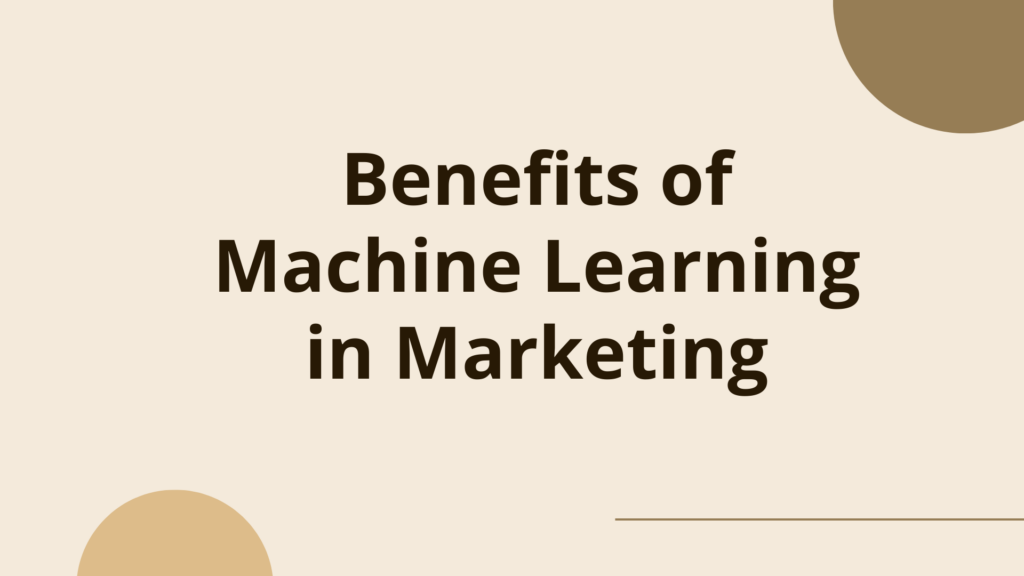
Increased Efficiency:
Machine learning can automate many marketing tasks, such as data analysis and customer segmentation, which can save time and reduce costs. This can enable marketers to focus on tasks like strategy development and creative content creation.
Enhanced Customer Experience:
Machine learning enables marketers to provide personalized and relevant experiences to customers, which can improve customer satisfaction and loyalty. By analyzing customer data, machine learning algorithms can identify individual preferences, interests, and behaviours and provide targeted recommendations and offers. This can result in increased engagement and higher conversion rates.
Improved Decision-Making:
Machine learning can provide marketers with valuable insights and predictions based on large volumes of data, which can inform marketing strategies and tactics. By leveraging machine learning algorithms, marketers can make more informed decisions about which campaigns to run, which channels to use, and which customers to target.
Higher Revenue and ROI:
Machine learning can help marketers to optimize their campaigns for maximum impact and return on investment (ROI). By using data-driven insights to inform campaign decisions, marketers can create more effective campaigns that drive higher revenue and ROI.
Competitive Advantage:
By leveraging machine learning in marketing, businesses can gain a competitive advantage by providing superior customer experiences, improving operational efficiency, and driving revenue growth. As machine learning becomes increasingly prevalent in marketing, businesses that do not adopt these technologies risk falling behind their competitors.
Challenges and Limitations of Machine Learning in Marketing
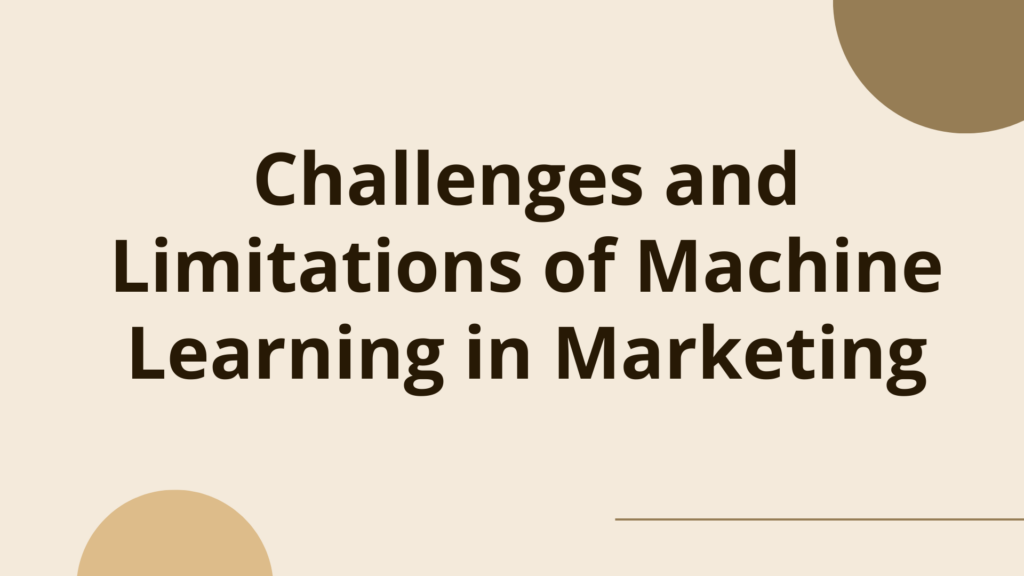
Data Quality and Quantity:
One of the biggest challenges of implementing machine learning in marketing is the quality and quantity of data available. Machine learning algorithms require large amounts of data to train effectively, and the quality of that data can impact the accuracy and effectiveness of the algorithms. Inaccurate or incomplete data can lead to biased or inaccurate results, impacting marketing decisions.
Interpretability and Explainability:
Machine learning algorithms can be complex and difficult to interpret and explain, making it challenging for marketers to understand how they arrive at their conclusions. This lack of interpretability can make it difficult for marketers to trust the insights and recommendations provided by machine learning algorithms.
Integration with Existing Systems:
Integrating machine learning systems with existing marketing systems can be a significant challenge. Legacy systems may not be compatible with newer technologies, making it difficult to share data or results between systems. This can create silos of data and insights, which can hinder the ability of marketers to make informed decisions.
Ethics and Privacy Concerns:
As machine learning algorithms become increasingly powerful and sophisticated, there are growing concerns about their impact on privacy and ethics. Machine learning algorithms may use personal data to make predictions and recommendations, which can raise concerns about data privacy and security. There is also a risk of algorithmic bias, perpetuating societal biases and discrimination.
Human Expertise:
Despite the advances in machine learning, human expertise and intuition remain essential in marketing. Machine learning algorithms can provide valuable insights and recommendations, but it is ultimately up to human marketers to interpret those insights and make decisions based on them. Marketers must balance relying on machine learning algorithms and leveraging their expertise and creativity.
Best Practices for Implementing Machine Learning in Marketing
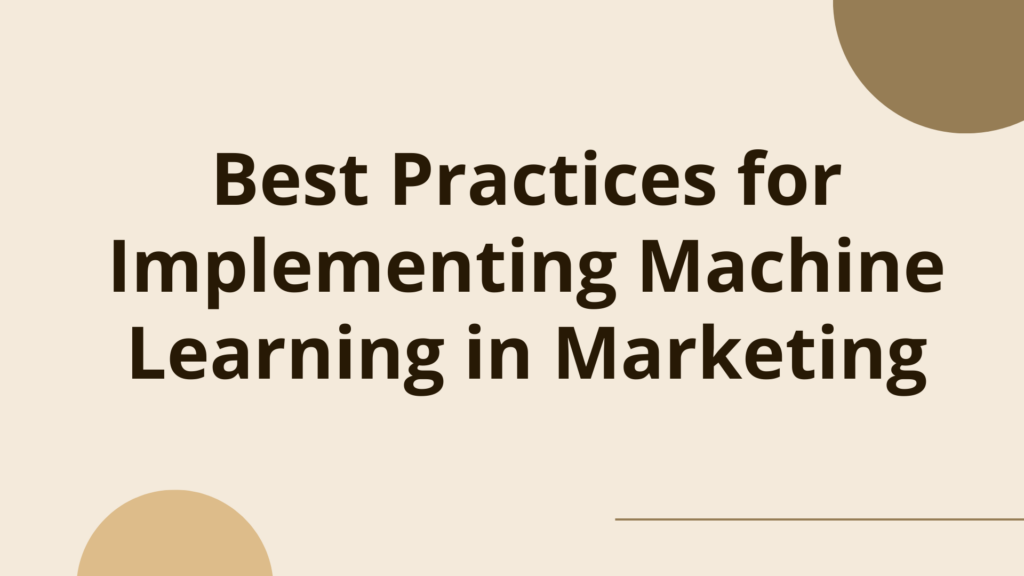
Define Clear Objectives:
Before implementing machine learning in marketing, it’s essential to define clear objectives and identify the problems that need solving. This can help ensure that the machine learning models are focused on the right areas and are aligned with business goals.
Gather Quality Data:
High-quality data is essential for machine learning models to perform effectively. It’s important to ensure the data used for training the models is accurate, complete, and relevant to the problem. This may involve cleaning and preprocessing the data to remove errors and inconsistencies.
Choose Appropriate Algorithms and Techniques:
A wide variety of machine learning algorithms and techniques are available, and it’s important to choose the appropriate ones based on the problem being addressed. This may involve evaluating the strengths and weaknesses of different algorithms and experimenting with different techniques to find the best fit.
Test and Validate Models:
Before deploying machine learning models in marketing campaigns, testing and validating them to ensure they perform as expected is important. This may involve using historical data to evaluate the accuracy of the models and fine-tune them for optimal performance.
Monitor and Update Models:
Machine learning models require ongoing monitoring and maintenance to ensure that they continue to perform effectively. This may involve regularly retraining the models with new data and updating them to reflect changes in the marketing environment.
Collaborate Across Teams:
Implementing machine learning in marketing requires collaboration across different teams and departments. Marketers, data scientists, and IT professionals must work together to ensure that the machine learning models are integrated with existing marketing systems and that insights and recommendations are effectively communicated to the broader marketing team.
Conclusion
In conclusion, machine learning has become essential for marketers looking to improve their efficiency, enhance customer experience, and increase revenue. By leveraging machine learning algorithms, marketers can personalize their marketing campaigns, segment their customers, and make data-driven decisions based on predictive analytics. However, challenges and limitations are associated with implementing machine learning in marketing, such as data quality and privacy concerns. To overcome these challenges, following best practices such as defining clear objectives, gathering quality data, choosing appropriate algorithms, testing and validating models, monitoring and updating models, and collaborating across teams is important. By doing so, marketers can maximize the benefits of machine learning and gain a competitive advantage in the rapidly evolving marketing landscape.
Leave a Reply